Diploma in Machine Learning with Python
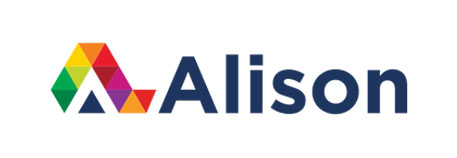
Alison Review
Location
Online(Course Link)
Dates
On Demand
Course Categories
IT, Technology and Software
Certficate
Yes()
Language
English
Course Fees
FreeNo. of Attendant
Unlimited
Acquired Skills/Covered Subjects
- Define machine learning
- Explain feature scaling and data cleaning
- Outline feature engineering
- Distinguish between parametric and non-parametric models
- Discuss the exploratory data analysis on the Iris dataset
Provider Name | Alison |
---|---|
Training Areas |
|
Website | https://alison.com/ |
About The Provider |
Alison is one of the world’s largest free
learning platforms for education and skills training. It is a for-profit social
enterprise dedicated to making it possible for anyone, to study anything,
anywhere, at any time, for free online, at any subject level. Through our
mission we are a catalyst for positive social change, creating opportunity,
prosperity, and equality for everyone. Alison was founded in Galway, Ireland, in 2007
and has grown organically to become a major force in free online education and
skills training. Today, with more than 13 million learners in 195 countries,
Alison is changing how the world learns and up-skills. They are committed to equality and access to
education and skills training irrespective of gender, geography, economic
status or any other barriers that can so often stunt potential. So they offer a
range of free courses that meet the many diverse needs of our learners. The UN
declared in Article 26 of the 1946 Declaration of Human Rights that “Education
shall be free…”. This statement will always inspire us. Alison was founded by Alison CEO, Mike Feerick. Mike is a businessman, but one with a
difference. He believes in social impact, and that you can build a financially
successful business focused on meeting a huge global social need, making
education and skills training more accessible for everyone. He invites anyone
who believes that too, to support the Alison mission.
Alison is free of charge to you. But it’s still
a business – albeit a socially-focused one. We are a social enterprise making
our money through advertising, merchandise, and the sale of Certificates and
Diplomas, should a graduate choose to buy one. |
with the world. Your Alison Diploma is:
Ideal for sharing with potential employers - include it in your CV, professional social media profiles and job applications
An indication of your commitment to continuously learn, upskill and achieve high results
An incentive for you to continue empowering yourself through lifelong learning
Alison offers 3 types of Diplomas for completed Diploma courses:
Digital Diploma - a downloadable Diploma in PDF format, immediately available to you when you complete your purchase
Diploma - a physical version of your officially branded and security-marked Diploma, posted to you with FREE shipping
Framed Diploma - a physical version of your officially branded and security-marked Diploma in a stylish frame, posted to you with FREE shipping
All Diplomas are available to purchase through the Alison Shop. For more information on purchasing Alison Diplomas, please visit our FAQs. If you decide not to purchase your Alison Diploma, you can still demonstrate your achievement by sharing your Learner Record or Learner Achievement Verification, both of which are accessible from your Dashboard. For more details on our Diploma pricing, please visit our Pricing Page.
This diploma course in machine learning with Python highlights the many benefits that Python has in its simplicity in machine learning and the frameworks of artificial intelligence (AI). Machine learning is a specific branch of artificial learning that is associated with computer science. Machine learning is about making software applications predict outcomes more accurately without any prior explicit programming. It provides computers with the capacity to learn without being obviously programmed. This course will cover two types of machine learning: supervised machine learning and unsupervised machine learning. Linear regression used to solve a regression problem is an excellent example of supervised machine learning. On the other hand, highlighting various customer groups to strategise marketing is a practical example of unsupervised learning. Within these two types of machine learning, various models depend on the purpose you would like to achieve. For instance, there are parametric models and non-parametric models, as covered in this course.
As you work through the content, you will determine that you resort to a parametric model when you precisely know which model you will fit to the data. Conversely, in a non-parametric model, the data directs you to what the regression should look like. For instance, the Key Nearest Neighbours (KNN) is a non-parametric method that you use for classification and regression. The KNN has led to a wider variety of applications, particularly in text mining, agriculture and finance. As with any modelling system, the KNN has its pros and cons. You will notice that the KNN is very easy to explain, simple to understand, and extremely powerful. Furthermore, KNN does not require any assumptions on the data distribution nor request any prior knowledge. However, the efficiency of the algorithm declines very quickly as the dataset grows. Moreover, KNN cannot work if there are any missing values.
Another crucial theme covered is the use of algorithms. Many algorithms will be unravelled throughout this course, each of which, as models, has its advantages and disadvantages. In this course, you are taught about the Random Forest. It is a slightly modified bagging algorithm, a collection of a decorrelated decision tree. The random forest is used because it works well with non-linear data, lowers the risk of overfitting and runs efficiently on large datasets. Conversely, it is biased while dealing with categorical variables. Thus, it is far from being suitable for linear methods with a lot of sparse features. Other methods, such as models and related algorithms, using Python as the backdrop programming language, are also delved into. This course is a windfall to students and professionals who are already familiar with Python and would like to become an expert in the field of machine learning.